On this planet of machine studying, we obsess over mannequin architectures, coaching pipelines, and hyper-parameter tuning, but usually overlook a elementary side: how our options stay and breathe all through their lifecycle. From in-memory calculations that vanish after every prediction to the problem of reproducing actual characteristic values months later, the way in which we deal with options could make or break our ML methods’ reliability and scalability.
Who Ought to Learn This
- ML engineers evaluating their characteristic administration strategy
- Knowledge scientists experiencing training-serving skew points
- Technical leads planning to scale their ML operations
- Groups contemplating Function Retailer implementation
Beginning Level: The invisible strategy
Many ML groups, particularly these of their early levels or with out devoted ML engineers, begin with what I name “the invisible strategy” to characteristic engineering. It’s deceptively easy: fetch uncooked knowledge, remodel it in-memory, and create options on the fly. The ensuing dataset, whereas purposeful, is basically a black field of short-lived calculations — options that exist just for a second earlier than vanishing after every prediction or coaching run.
Whereas this strategy might sound to get the job performed, it’s constructed on shaky floor. As groups scale their ML operations, fashions that carried out brilliantly in testing immediately behave unpredictably in manufacturing. Options that labored completely throughout coaching mysteriously produce completely different values in stay inference. When stakeholders ask why a particular prediction was made final month, groups discover themselves unable to reconstruct the precise characteristic values that led to that call.
Core Challenges in Function Engineering
These ache factors aren’t distinctive to any single staff; they symbolize elementary challenges that each rising ML staff ultimately faces.
- Observability
With out materialized options, debugging turns into a detective mission. Think about making an attempt to grasp why a mannequin made a particular prediction months in the past, solely to search out that the options behind that call have lengthy since vanished. Options observability additionally allows steady monitoring, permitting groups to detect deterioration or regarding traits of their characteristic distributions over time. - Cut-off date correctness
When options utilized in coaching don’t match these generated throughout inference, resulting in the infamous training-serving skew. This isn’t nearly knowledge accuracy — it’s about guaranteeing your mannequin encounters the identical characteristic computations in manufacturing because it did throughout coaching. - Reusability
Repeatedly computing the identical options throughout completely different fashions turns into more and more wasteful. When characteristic calculations contain heavy computational assets, this inefficiency isn’t simply an inconvenience — it’s a major drain on assets.
Evolution of Options
Method 1: On-Demand Function Technology
The best resolution begins the place many ML groups start: creating options on demand for quick use in prediction. Uncooked knowledge flows by means of transformations to generate options, that are used for inference, and solely then — after predictions are already made — are these options sometimes saved to parquet information. Whereas this technique is simple, with groups usually selecting parquet information as a result of they’re easy to create from in-memory knowledge, it comes with limitations. The strategy partially solves observability since options are saved, however analyzing these options later turns into difficult — querying knowledge throughout a number of parquet information requires particular instruments and cautious group of your saved information.
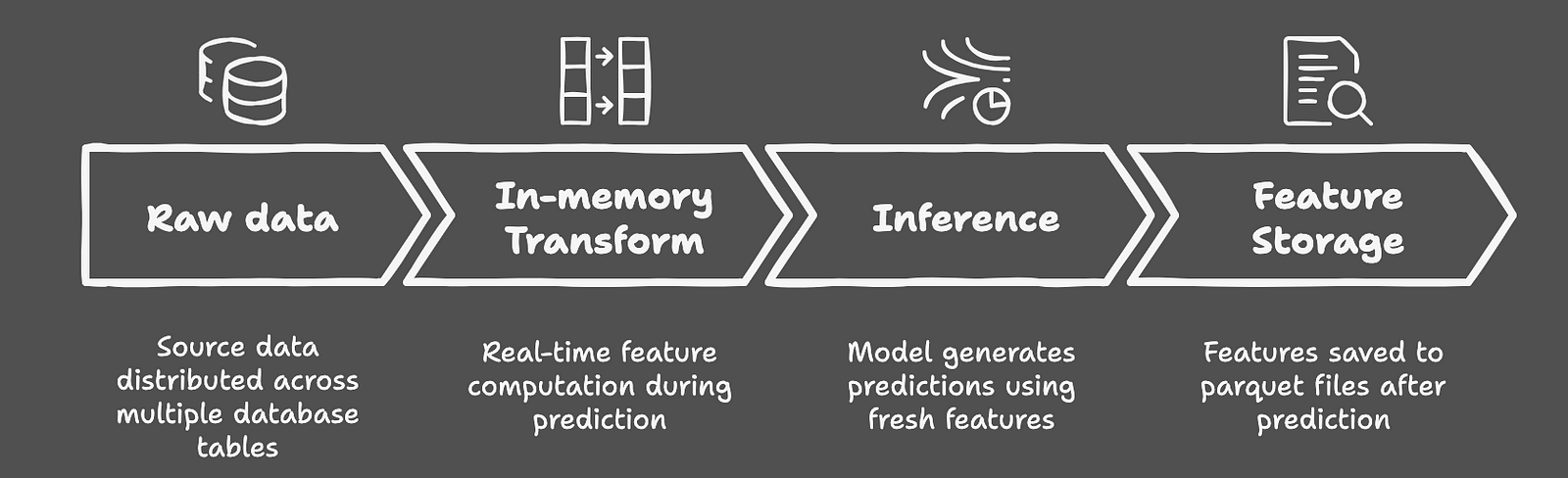
Method 2: Function Desk Materialization
As groups evolve, many transition to what’s generally mentioned on-line as an alternative choice to full-fledged characteristic shops: characteristic desk materialization. This strategy leverages present knowledge warehouse infrastructure to rework and retailer options earlier than they’re wanted. Consider it as a central repository the place options are constantly calculated by means of established ETL pipelines, then used for each coaching and inference. This resolution elegantly addresses point-in-time correctness and observability — your options are all the time obtainable for inspection and constantly generated. Nevertheless, it exhibits its limitations when coping with characteristic evolution. As your mannequin ecosystem grows, including new options, modifying present ones, or managing completely different variations turns into more and more advanced — particularly resulting from constraints imposed by database schema evolution.
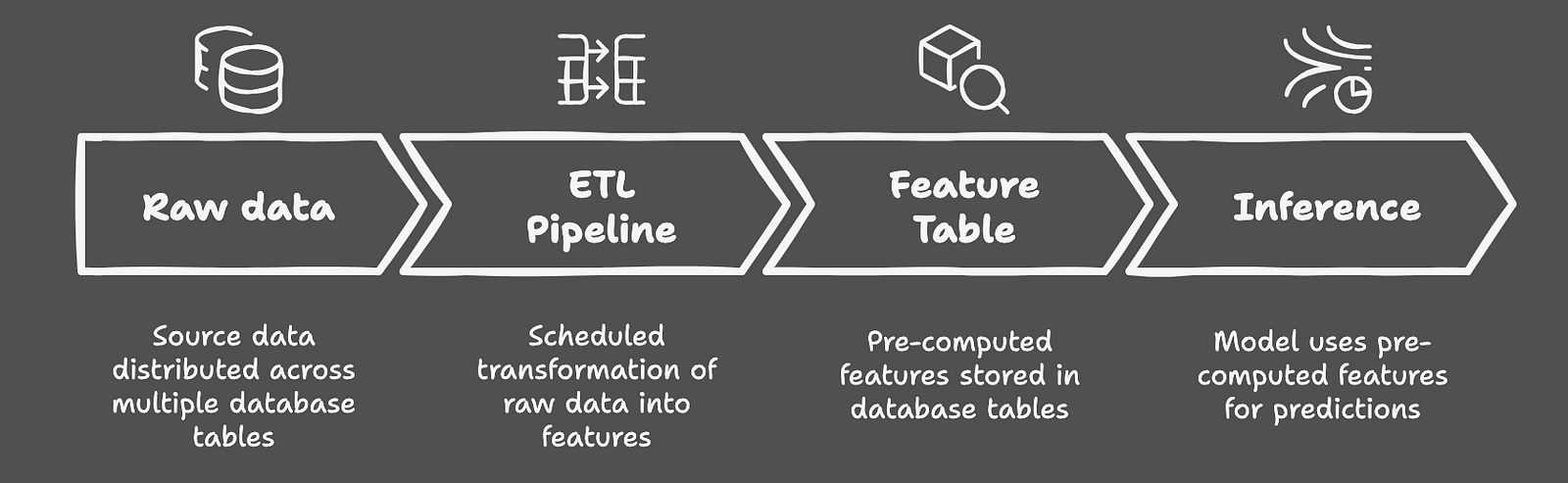
Method 3: Function Retailer
On the far finish of the spectrum lies the characteristic retailer — sometimes a part of a complete ML platform. These options provide the complete package deal: characteristic versioning, environment friendly on-line/offline serving, and seamless integration with broader ML workflows. They’re the equal of a well-oiled machine, fixing our core challenges comprehensively. Options are version-controlled, simply observable, and inherently reusable throughout fashions. Nevertheless, this energy comes at a major value: technological complexity, useful resource necessities, and the necessity for devoted ML Engineering experience.
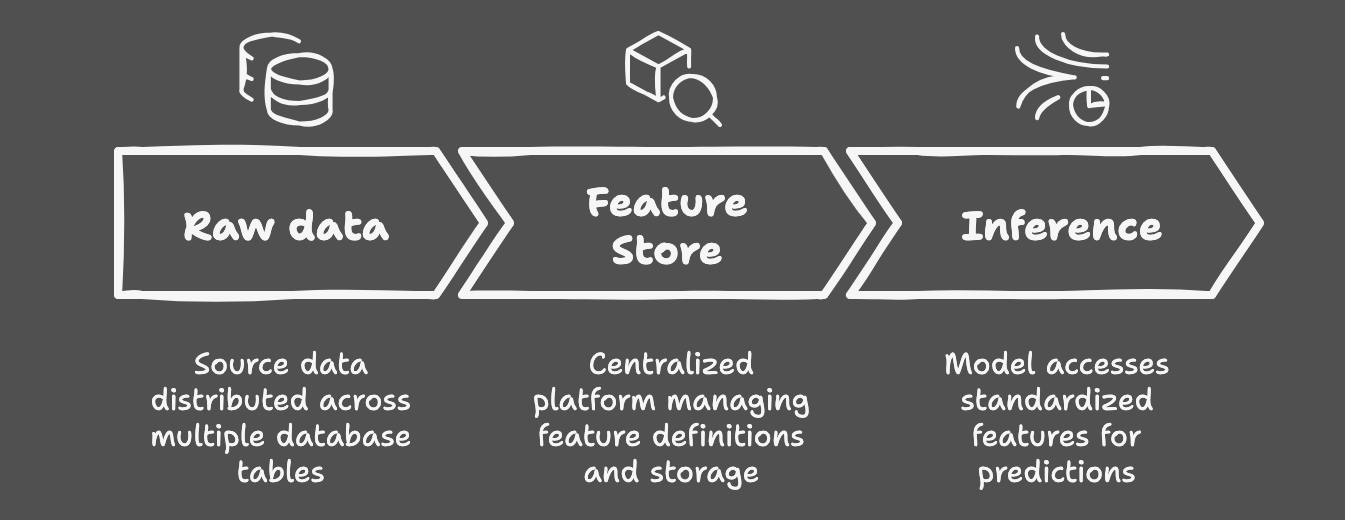
Making the Proper Selection
Opposite to what trending ML weblog posts may counsel, not each staff wants a characteristic retailer. In my expertise, characteristic desk materialization usually offers the candy spot — particularly when your group already has sturdy ETL infrastructure. The secret’s understanding your particular wants: should you’re managing a number of fashions that share and steadily modify options, a characteristic retailer is likely to be well worth the funding. However for groups with restricted mannequin interdependence or these nonetheless establishing their ML practices, easier options usually present higher return on funding. Positive, you might keep on with on-demand characteristic technology — if debugging race situations at 2 AM is your thought of time.
The choice in the end comes all the way down to your staff’s maturity, useful resource availability, and particular use instances. Function shops are highly effective instruments, however like all subtle resolution, they require vital funding in each human capital and infrastructure. Generally, the pragmatic path of characteristic desk materialization, regardless of its limitations, affords the very best stability of functionality and complexity.
Keep in mind: success in ML characteristic administration isn’t about selecting probably the most subtle resolution, however discovering the fitting match on your staff’s wants and capabilities. The secret’s to actually assess your wants, perceive your limitations, and select a path that allows your staff to construct dependable, observable, and maintainable ML methods.