Supercharging AI with a Complete Knowledge Catalog and Strong Entry Controls
As knowledge grows in quantity, AI turns into more and more very important for analytical duties inside organizations. Nevertheless, for AI to offer dependable and significant insights, it have to be constructed with a complete understanding of this knowledge.
As well as, efficient knowledge entry controls have to be deployed to make sure that knowledge stays accessible but safe. These parts guarantee a powerful basis for AI instruments that may vastly increase a company’s analytical capabilities whereas concurrently making certain the accountable use of AI.
Know Your Knowledge
There are various ways in which AI may be utilized to deal with a company’s wants. One highly effective utility is how AI-powered access-controlled knowledge catalogs can allow companies to generate stories with out requiring deep technical information. These stories are context-aware, correct, and designed to satisfy particular entry ranges. AI may also be utilized to suggest the perfect datasets for particular tasks primarily based on entry constraints, addressing venture wants whereas making certain compliance to safety tips. One other utility lies in AI’s capability to research ETL code, which may present clear lineage monitoring for knowledge high quality assessments by providing insights into knowledge transformations, origins, and circulate.
Nevertheless, for these instruments to be efficient, they require an in depth understanding of the info they function on. A complete knowledge catalog contains not solely the uncooked knowledge but in addition metadata, knowledge lineage, and annotations from material consultants. Metadata—resembling column names, knowledge sorts, and measurement models—allows AI instruments to interpret and analyze knowledge precisely. Knowledge lineage gives info on the origin of every dataset, any transformations utilized, and integrations with different datasets, providing useful context past metadata alone. Monitoring knowledge lineage via complicated ETL (Extract, Rework, Load) processes is important to offer this layer of transparency, however may be difficult to offer. Lastly, professional notes and annotations contribute further insights that assist AI perceive the info from a domain-specific perspective. Alongside the catalog, knowledge entry controls be sure that AI instruments can function inside safe and compliant boundaries, permitting contextual evaluation whereas safeguarding knowledge privateness.
We’ll present an instance of those parts by analyzing a knowledge catalog of healthcare data. On this situation, metadata may describe affected person demographics and medical historical past knowledge sorts, enabling AI to interpret every area appropriately. Knowledge lineage traces the info’s journey from scientific data to analytical dashboards, preserving important context about every transformation. Professional annotations, resembling clinician insights or diagnostic notes, enrich this context, serving to AI distinguish between related medical phrases or circumstances. Lastly, entry controls limit the info and use of corresponding AI instruments to licensed customers, making certain knowledge privateness and regulatory compliance. This built-in strategy improves the accuracy and reliability of AI-driven insights in a delicate area.
Construct an Efficient Knowledge Catalog with Entry Controls
To construct a knowledge catalog that helps efficient AI use whereas sustaining strict safety, it’s important to observe a structured strategy that enriches knowledge, tracks its origins, integrates professional insights, and controls entry. The next steps define the beneficial practices to realize a strong and dependable knowledge catalog:
1. Metadata Enrichment: Guarantee every dataset is supplied with full metadata, together with knowledge sorts, models, and descriptions. Enrich metadata with standardized tags and detailed descriptions to enhance AI’s interpretability and facilitate knowledge discovery throughout the catalog.
2. Lineage Documentation: Preserve exact knowledge lineage to trace the origin, transformations, and interactions of datasets. Superior AI-driven brokers can analyze ETL scripts on to hint lineage via every step and make sure the reliability of the info. For an in-depth dialogue on this subject, discuss with our earlier weblog submit on utilizing AI to trace lineage in ETL pipelines.
3. Professional Annotations: Combine annotations from material consultants so as to add contextual insights that enrich datasets. Select instruments that help collaborative knowledge cataloging, permitting consultants to contribute information instantly inside the catalog. Annotation capabilities present AI with domain-specific context, growing the relevance and reliability of analyses.
4. Entry Management Mechanisms: Implement exact entry permissions to make sure knowledge availability solely to licensed customers. Tremendous-tuned entry settings be sure that delicate knowledge is accessible solely to these with applicable permissions, minimizing danger whereas supporting knowledge governance.
Utilizing these methods to boost knowledge cataloging and management entry strengthens knowledge governance, making certain the catalog is each safe and optimized for efficient AI use.
Conclusion
A complete knowledge catalog with sturdy entry management, complemented by professional insights, is important for safe and efficient AI-driven knowledge administration. By prioritizing these components, organizations can empower AI techniques to generate exact insights, automate reporting, and suggest knowledge confidently.
Concerning the Writer
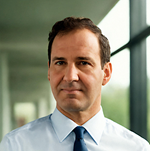
John Mark Suhy is CTO of Greystones Group. Mr. Suhy brings greater than 20 years of enterprise structure and software program improvement expertise with main companies together with FBI, Sandia Labs, Division of State, US Treasury and the Intel group. Mr. Suhy authored the Authorities Version of Neo4j, the world’s main graph database supporting Synthetic Intelligence/Machine Studying and Pure Language Processing. He is also the co-founder of the open supply ONgDB and DozerDb graph database tasks. Mr. Suhy is a frequent speaker at prestigious occasions resembling RSA. He holds a B.S. in Laptop Science from George Mason College in Virginia.
Join the free insideAI Information publication.
Be part of us on Twitter: https://twitter.com/InsideBigData1
Be part of us on LinkedIn: https://www.linkedin.com/firm/insideainews/
Be part of us on Fb: https://www.fb.com/insideAINEWSNOW
Test us out on YouTube!